Identifying the most important spectral and textural features for mapping specific crops using very high spatial resolution data
Objective:
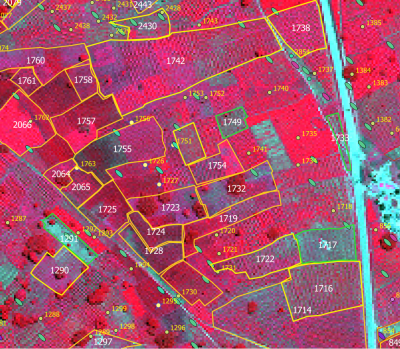
Description:
This MSc topic is linked to the STARS (Spurring a Transformation for Agriculture through Remote Sensing) project. STARS explores ways to use remote sensing technology to improve agricultural practices of smallholder farmers in sub-Saharan Africa and South Asia with the hope of advancing the livelihoods of famers and their families in some of the world’s poorest countries.
To achieve this, STARS has collected a temporally dense set of RGB and multispectral images over agricultural lands dominated by smallholder farming activities. These images, acquired from unmanned aerial vehicles (UAVs) as well as from very high spatial resolution (VHR) satellite sensors, were used to recognize crop types and to monitor crop condition. This topic deals with the former task. More precisely, with the use of STARS images to map a specific crop or cropping system. For this task, we propose to use one-class classification methods.
One-class classification methods are a subset of machine learning methods that try to recognize specific objects (in this case: a specific crop or cropping systems) by learning from a training set composed of objects of that class (in this case, pixels labelled as crop/cropping system X during field work). A wide range of one-class classification methods exists (see Tax, 2001 for a review).
The work for this MSc topic will start with a literature review to identify the most promising methods. Then the student will select one or more crops monitored by STARS and one or more areas (e.g. Mali, Nigeria, Tanzania). After that, a comprehensive assessment of the performance of the chosen one-class classification methods will be done by paying special attention to the spectral or textural features that are key for a successful classification. The STARS Crop Spectrotemporal Signature Library (CSSL), which contains spectral, textural, environmental and crop-related information could be used to quickly train and evaluate different classification methods.
Given the nature of the topic, an analytical mind and affinity with scripting are highly recommended for this MSc research topic. For more information on the STARS project visit: http://www.stars-project.org
References:
Désir, C., S. Bernard, C. Petitjean, and L. Heutte, One class random forests. Pattern Recognition, 2013. 46(12): p. 3490-3506.
Tax, D., One-class classification: concept-learning in the absence of counter-examples. 2001 (http://homepage.tudelft.nl/n9d04/thesis.pdf)