Designing operational data-driven forecasts models
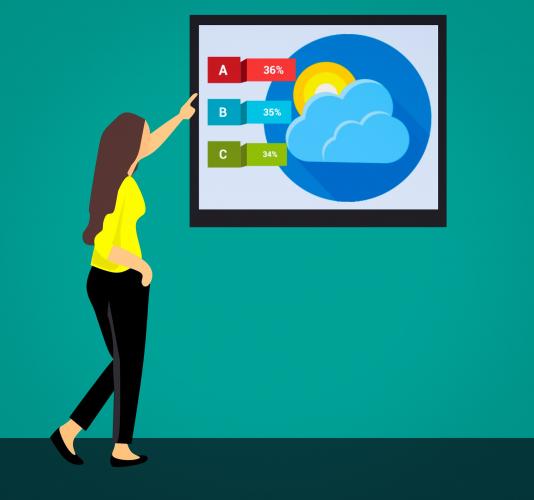
Objective:
Keywords:
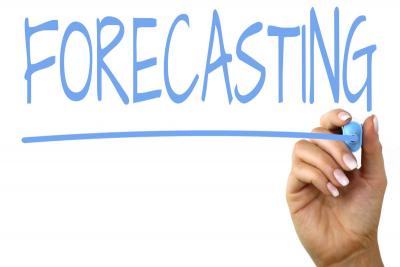
Description:
Data-driven models are becoming more and more common thanks to the increasing availability of big geo-datasets and computational power and intelligence. Many of these models have a strong exploratory or explanatory character. Yet, I believe that a pre-requisite to advance our scientific knowledge is to shift from only observing and monitoring to designing models that can anticipate change, and timely inform decision-makers and other stakeholders. For practical purposes, these models should not only be robust and accurate but also operational. Hence, this MSc topic deals with the design of operational data-driven forecasts models.
A topic that is particularly suited for designing data-driven forecasts models is phenology. Phenology is the science that supports vegetation seasonality studies. Phenology focuses on studying the timing of recurring biological phases, their causes, and their interrelations. The timing of these phases varies from year to year and from place to place because phenology is strongly influenced by weather and climatic variability. Here we propose using operational short-term weather forecasts (available as raster layers) to run various phenological models that predict spring onset. This will allow us to forecast the timing of spring events a5 to 10 days before they happen. Since weather forecasts often come as ensembles, we can run our models on each ensemble member to estimate the uncertainty of our forecasts.
The modeling work will involve using machine learning regression methods. The operationalization of the models will require building an information infrastructure to get model inputs, run the models and display the output maps using web GIS technology.
References:
I. Ibáñez et al., “Forecasting phenology under global warming,” Philos. Trans. R. Soc. B-Biological Sci., vol. 365, no. 1555, pp. 3247–3260, 2010.
M. C. Dietze et al., “Iterative near-term ecological forecasting: Needs, opportunities, and challenges.,” Proc. Natl. Acad. Sci. USA, vol. 115, no. 7, pp. 1424–1432, Feb. 2018